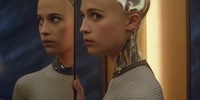
Background information
Artificial intelligence - when is it considered intelligent?
by Kevin Hofer
These days, artificial intelligence and machine learning are often uttered in the same breath. While machine learning is a branch of artificial intelligence, these terms aren’t synonymous. The same goes for deep learning. Here’s my attempt to set them apart once and for all.
Let me give you an example. The time had come round again for me to get my hair cut. That would mean making an appointment at the hairdresser’s. Unfortunately, my stylist doesn’t take online bookings so I’d have to ring up. Now, using the phone is not something I enjoy at all. Thankfully, that’s a job I could ask Google to do.
This shows quite clearly what AI is. In terms of hierarchy, artificial intelligence is the umbrella term for talking about machine intelligence. You might not be aware of it but you probably encounter artificial intelligence in everyday life. Google Assistant is a prime example.
How do you know if something harnesses artificial intelligence. Well, AI should be able to plan, navigate, process language, come to logical conclusions and understand and interact with the world. It’s also supposed to possess knowledge representation, emotional intelligence and morals.
Machine learning is a branch of artificial intelligence and in turn deep learning is a branch of machine learning. But what’s the story with these terms?
An example of machine learning is Netflix’s recommendation system. It’s an integral component of this streaming service. Based on your preferences and feedback, Netflix recommends films, series and documentaries it thinks you might like.
Machine learning works based on a system that’s fed with data and information. Take traffic, for example. Say I want to know how many vehicles drive past my house in the afternoons. Imagine also that I’d like to sort them by vehicle type. I could count them myself but that’d be way too time-consuming.
Instead I feed a machine learning system with visual data on different vehicles (cars, bikes, motorbikes). In addition, I give the system info on the characteristics of these vehicles. By that I mean things like the fact a bicycle normally has two wheels, pedals and handlebars. The system then learns to distinguish these vehicles using visual characteristics.
Once I’m done feeding in all the available data, I let the system’s eyes loose on the street. This allows it to keep picking up new data and matching it up with information stored. And that, my friends, is what reveals the volume and type of traffic going past my house.
With machine learning, the system can make predictions based on established data. Granted, the system does need data to kick things off and to help it learn but it doesn’t require as much as a deep learning system does. This makes machine learning appropriate for simpler systems. You just need to be aware that most data has to be input from the outset. Tasks then get segmented and split into separate parts.
It was quite a while before I knew if the system was going to be smart enough. You see, the test phase is rather long. On the plus side, the system is easy to understand as the rules are man-made.
You can see deep learning in practice in the automatic toning of black-and-white photos. A deep learning system learns from colour patterns you tend to find on photos – like the sky usually being blue and clouds often looking whitish grey. It then applies this knowledge to other black-and-white photos.
Unlike in traditional machine learning, systems using deep learning can also learn from themselves. In unmonitored deep learning, data is still fed in by hand, but the system processes this itself using artificial neuronal networks.
Let’s go back to the vehicle counting example from before. This time the system is given visual data without any additional information. The system separates these images into coarse pixels (e.g. 28×28). Afterwards, information passes through the artificial neuronal network so the system learns to distinguish different types of vehicles. Once it has learnt that, we set new data loose on the system. In fact, when we input the traffic count, the system picks it all up and scores top marks.
How the whole thing works in details is quite mind-boggling. I’m battling with the concept at the moment and will soon be putting pen to paper (or rather fingers to keyboard).
In the meantime, let me explain it this way. Deep learning systems think and learn thanks to artificial neuronal networks, just as humans do. Performance is enhanced the more data the system has in its reach. Deep learning systems need a fair amount of data – much more so than machine learning equivalents do. The thing to remember is that this branch of machine learning offers more scalability than other ML systems.
But that does come at a price. Deep learning fairly glugs down computing power, which is why you usually find it in complex systems. Tasks are recorded in their entirety and the system decides on its own what it learns from. While it does take deep learning systems longer than other ML systems to learn, they don’t need to spend as much time in the test phase.
Got all that? Here’s a synopsis of everything we covered: AI is a concept and machine learning is one way to achieve AI. You can think of artificial intelligence as the container or vessel. Within that, intelligent machines think and act like people. In machine learning, systems learn through programming, while in deep learning, the system can also learn for itself.
Put another way, all machine learning is AI but not all AI is machine learning. Similarly, all deep learning is machine learning but not all machine learning is deep learning. And last but not least, all deep learning is AI but not all AI is deep learning.
Don’t worry if your head feels like it’s buzzing. It’s quite simple. Honest.
From big data to big brother, Cyborgs to Sci-Fi. All aspects of technology and society fascinate me.